Our Free Services
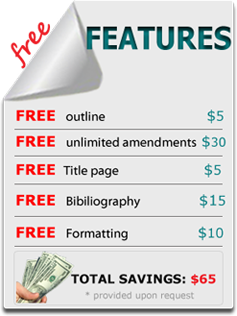
Paper Formatting
- Double or single-spaced
- 1-inch margin
- 12 Font Arial or Times New Roman
- 300 words per page
No Lateness!

Our Guarantees
- Free Unlimited revisions
- Guaranteed Privacy
- Money Return guarantee
- Plagiarism Free Writing
Generative AI and how is it similar/different to Traditional AI?
- What is Generative AI and how is it similar/different to Traditional AI?
- Do you believe that work created by Generative AI (e.g. ChatGPT) is comparable in quality to human created content? What challenges and opportunities does Generative AI pose to cyber security?
Sample Answer
1. Generative AI vs. Traditional AI
Generative AI is a subfield of artificial intelligence focused on creating new, original content that resembles the data it was trained on. This content can take various forms, including text, images, audio, video, and even code. The core principle behind generative AI is learning the underlying patterns and structures within a dataset and then using that knowledge to produce novel instances that adhere to those patterns. Prominent examples include large language models (LLMs) like ChatGPT that generate human-like text, diffusion models like DALL-E 2 and Stable Diffusion that create images from text prompts, and models that can generate music or even design molecules for drug discovery.
Full Answer Section
Traditional AI, in contrast, is typically focused on tasks like classification, prediction, and pattern recognition within existing data. It excels at analyzing data to identify categories (e.g., classifying emails as spam or not spam), forecasting future outcomes (e.g., predicting stock prices or customer churn), and finding correlations or anomalies in data (e.g., identifying fraudulent transactions). Traditional AI models are often designed to provide a specific output based on a given input, following a more deterministic or rule-based approach, even if the rules are learned from data.
Here’s a breakdown of their similarities and differences:
Similarities:
- Relies on Data: Both generative and traditional AI heavily depend on large datasets for training. The quality and quantity of the training data significantly impact the performance of both types of AI.
- Uses Machine Learning: Both leverage machine learning algorithms to learn from data. This includes various techniques like supervised learning, unsupervised learning, and reinforcement learning, although the specific applications and architectures may differ.
- Aims for Automation and Problem Solving: Ultimately, both generative and traditional AI aim to automate tasks, solve problems, and provide valuable insights or outputs.
- Requires Computational Resources: Training and running both types of AI models often demand significant computational power, especially for complex tasks and large models.
- Potential for Bias: Both are susceptible to biases present in their training data, which can lead to skewed or unfair outputs.
Differences:
Feature | Generative AI | Traditional AI |
---|---|---|
Primary Goal | Create new, original content | Analyze, classify, predict, or recognize patterns in existing data |
Output | Novel instances of data (text, images, etc.) | Labels, predictions, classifications, scores |
Nature of Output | Open-ended, creative, can be highly variable | More structured, often a specific answer or category |
Learning Focus | Learning the underlying distribution and structure of data to generate new samples | Learning the mapping between inputs and predefined outputs or identifying patterns within the data |
Examples | ChatGPT, DALL-E 2, Stable Diffusion, music generators | Image recognition software, spam filters, fraud detection systems, recommendation engines |
Evaluation | Often involves subjective measures (e.g., coherence, realism) and statistical metrics (e.g., perplexity, Inception Score) | Typically evaluated using objective metrics (e.g., accuracy, precision, recall, F1-score) |
Creativity/Novelty | Inherently designed to produce novel outputs | Primarily focused on accurate analysis of existing data |
Application Focus | Content creation, design, simulation, drug discovery | Automation, decision support, analysis, surveillance |
In essence, traditional AI is about understanding and working with existing data, while generative AI is about learning from existing data to create new data. The distinction, however, can sometimes blur as generative models are increasingly used for tasks like data augmentation (creating synthetic data to improve traditional AI model training) or for generating explanations (a form of text generation).
2. Quality of Generative AI Content and Cybersecurity Implications
Comparability of Generative AI Content to Human-Created Content:
The question of whether work created by generative AI is comparable in quality to human-created content is complex and highly dependent on the specific task, the capabilities of the AI model, and the criteria for “quality.”
Currently, generative AI can produce content that is often remarkably human-like in style and even substance. For tasks like generating basic factual summaries, drafting routine emails, creating simple code snippets, or producing visually appealing images based on prompts, generative AI can achieve output that is often indistinguishable from, or even surpasses, human capabilities in terms of speed and efficiency.
However, true parity in quality across all domains is still a significant challenge. Human creativity, critical thinking, nuanced understanding of context, emotional intelligence, and the ability to draw upon real-world experience and common sense often surpass the capabilities of current generative AI.
Here’s a more nuanced breakdown:
-
Strengths of Generative AI:
- Efficiency and Speed: Can generate large volumes of content quickly.
- Consistency in Style: Can maintain a consistent tone and style.
- Accessibility: Makes content creation tools available to a wider audience.
- Automation of Repetitive Tasks: Can automate mundane content creation tasks.
- Novel Combinations: Can sometimes generate unexpected and creative combinations of ideas.
- Efficiency and Speed: Can generate large volumes of content quickly.
-
Limitations of Generative AI:
- Lack of True Understanding: Operates based on patterns in data, not genuine comprehension.
- Potential for Inaccuracy and Bias: Can generate factually incorrect or biased content if the training data is flawed.
- Limited Creativity and Originality: While it can produce novel combinations, true originality often requires human insight and intention.
- Difficulty with Nuance and Context: May struggle with complex or subtle contexts, leading to outputs that are technically correct but lack deeper understanding.
- Ethical Concerns: Issues related to plagiarism, copyright, and the potential for misuse.
- Absence of Lived Experience and Emotion: Struggles to convey genuine emotion or draw upon personal experiences in a meaningful way.
- Lack of True Understanding: Operates based on patterns in data, not genuine comprehension.
In conclusion, while generative AI is rapidly advancing and can produce impressive content, it is not yet universally comparable in quality to human-created content, especially in tasks requiring deep understanding, critical thinking, genuine creativity, and emotional intelligence. The “quality” is highly context-dependent. Generative AI is often best seen as a powerful tool that can augment human creativity and productivity, rather than a complete replacement.
Challenges and Opportunities Generative AI Poses to Cybersecurity:
Generative AI presents a double-edged sword for cybersecurity, offering both significant opportunities for defense and creating novel and sophisticated threats.
Challenges:
- Sophisticated Phishing and Social Engineering: Generative AI can create highly realistic and personalized phishing emails, social media posts, and text messages that are much harder to detect than traditional attempts. These can be tailored to individual targets, leveraging publicly available information to build trust and increase the likelihood of success.
- Generation of Realistic Deepfakes: AI can generate increasingly convincing fake videos and audio (deepfakes) that can be used for disinformation campaigns, financial fraud (e.g., impersonating executives), and reputational damage.
- Automated Malware and Exploit Development: While still in early stages, generative AI could potentially be used to automate the creation of new and polymorphic malware that can evade traditional security defenses. It might also assist in identifying and exploiting software vulnerabilities more efficiently.
- Circumventing Security Controls: AI could be used to generate adversarial attacks that are specifically designed to bypass existing security systems, such as intrusion detection and prevention systems.
- Disinformation and Propaganda at Scale: Generative AI can create vast amounts of realistic-looking fake news articles, social media content, and propaganda, making it harder to distinguish truth from falsehood and potentially manipulating public opinion.
- Evasion of Content Moderation: Malicious actors can use generative AI to create harmful content that can adapt and evolve to evade content moderation algorithms.
Opportunities:
- Enhanced Threat Detection and Analysis: AI can analyze vast amounts of security data (logs, network traffic, etc.) to identify subtle patterns and anomalies that might indicate sophisticated attacks, potentially detecting threats that human analysts might miss.
- Automated Security Responses: Generative AI could be used to automate responses to cyber threats, such as isolating infected systems, blocking malicious traffic, and patching vulnerabilities more quickly and efficiently.
- Improved Security Awareness Training: AI can create personalized and engaging security awareness training materials, including realistic simulations of phishing attacks, to educate users and improve their ability to identify threats.
- Vulnerability Discovery and Patching: AI could assist in identifying software vulnerabilities by analyzing code and generating potential exploits, helping developers to proactively patch them.
- Development of More Robust Authentication and Authorization Systems: AI-powered biometric authentication and adaptive access control systems could enhance security by continuously assessing user behavior and context.
- Creation of Deeper Fake Detection Tools: Generative AI can also be used to develop more sophisticated tools for detecting deepfakes and other forms of AI-generated malicious content.
In conclusion, generative AI presents a significant paradigm shift in the cybersecurity landscape. While it offers powerful new tools for defenders, it also empowers attackers with the ability to create more sophisticated and adaptive threats. The cybersecurity community must proactively research, develop, and deploy AI-powered defenses to stay ahead of these evolving challenges. A constant arms race between offensive and defensive AI capabilities is likely to define the future of cybersecurity.
This question has been answered.
Get AnswerOur Services
- Research Paper Writing
- Essay Writing
- Dissertation Writing
- Thesis Writing
Daily Statistics
- 134 New Projects
- 235 Projects in Progress
- 432 Inquiries
- 624 Repeat clients
Why Choose Us
- Money Return guarantee
- Guaranteed Privacy
- Written by Professionals
- Paper Written from Scratch
- Timely Deliveries
- Free Amendments