Our Free Services
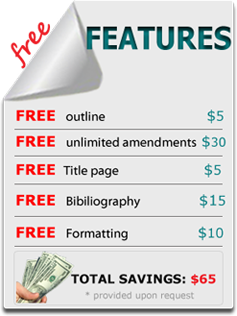
Paper Formatting
- Double or single-spaced
- 1-inch margin
- 12 Font Arial or Times New Roman
- 300 words per page
No Lateness!

Our Guarantees
- Free Unlimited revisions
- Guaranteed Privacy
- Money Return guarantee
- Plagiarism Free Writing
Enhancing Cyber Security In Healthcare -With The Help Of Machine Learning
Write a research paper than contains following:
- A brief discussion of the dissertation topic “Enhancing Cyber Security In Healthcare -With The Help Of Machine Learning” and why.
- The research question(s) you will use to employ to explore the topic.
- A brief discussion of how you plan to design your study to answer the research questions from #2 (above)
Sample Answer
Enhancing Cybersecurity in Healthcare with the Help of Machine Learning: A Research Proposal
1. Dissertation Topic Discussion and Rationale:
The proposed dissertation topic, “Enhancing Cybersecurity in Healthcare: Leveraging Machine Learning for Proactive Threat Detection and Response,” addresses a critical and increasingly urgent challenge within the healthcare sector. The healthcare industry, entrusted with highly sensitive patient data, including personal health information (PHI), financial details, and even genetic data, has become a prime target for cyberattacks. The consequences of these breaches can be devastating, ranging from financial losses and reputational damage to compromising patient safety and eroding trust in the healthcare system.
Full Answer Section
Several factors underscore the significance and timeliness of this research:
- High Value of Healthcare Data: PHI is significantly more valuable on the black market than other types of personal data due to its comprehensiveness and potential for identity theft, insurance fraud, and even blackmail. This high value makes healthcare organizations an attractive target for sophisticated cybercriminals.
- Increasing Sophistication of Cyber Threats: Cyberattacks are becoming increasingly complex, employing advanced techniques like ransomware, distributed denial-of-service (DDoS) attacks, and advanced persistent threats (APTs) that can evade traditional security measures. The healthcare sector, often characterized by legacy systems and interconnected devices, presents a vulnerable attack surface.
- Regulatory Compliance and Legal Ramifications: Healthcare organizations are subject to stringent regulations like HIPAA (in the US) and GDPR (in Europe), which mandate the protection of patient data. Security breaches can lead to severe legal penalties, financial repercussions, and loss of patient trust.
- Impact on Patient Care: Cyberattacks can disrupt healthcare operations, leading to appointment cancellations, delays in treatment, and even the inability to access critical patient information during emergencies. This can directly impact patient safety and well-being.
- Potential of Machine Learning in Cybersecurity: Machine learning (ML) offers promising avenues for enhancing cybersecurity defenses beyond traditional rule-based systems. ML algorithms can analyze vast amounts of data to identify patterns, anomalies, and subtle indicators of malicious activity that human analysts or static rules might miss. This proactive capability is crucial in detecting and responding to sophisticated and novel cyber threats in real-time.
Therefore, exploring the application of machine learning techniques to bolster cybersecurity within the healthcare sector is not merely an academic exercise but a necessity for safeguarding sensitive patient information, ensuring operational continuity, and ultimately protecting patient health and trust in the healthcare system. This research aims to contribute to a deeper understanding of how ML can be effectively implemented to create more resilient and adaptive cybersecurity defenses in this critical domain.
2. Research Question(s):
To thoroughly explore the potential of machine learning in enhancing healthcare cybersecurity, this dissertation will be guided by the following primary and secondary research questions:
Primary Research Question:
- How can machine learning techniques be effectively employed to enhance the proactive detection and response capabilities against major cybersecurity threats targeting healthcare organizations?
Secondary Research Questions:
- What are the specific types of cybersecurity threats that pose the most significant risks to healthcare organizations and their data?
- Which machine learning algorithms and models are most promising for identifying and predicting these specific cybersecurity threats in healthcare environments?
- What are the key challenges and limitations associated with implementing machine learning-based cybersecurity solutions in healthcare settings, including issues related to data privacy, regulatory compliance, and system integration?
- How can the performance and reliability of machine learning-based cybersecurity systems in healthcare be effectively evaluated and validated?
- What are the organizational and technical considerations for the successful adoption and integration of machine learning-driven cybersecurity measures within existing healthcare infrastructure and workflows?
3. Study Design:
To address the research questions outlined above, this study will employ a mixed-methods research design, combining both quantitative and qualitative data collection and analysis techniques. This approach will allow for a comprehensive understanding of the technical capabilities of machine learning in healthcare cybersecurity and the practical challenges and considerations for its implementation.
Phase 1: Literature Review and Threat Landscape Analysis (Qualitative):
This initial phase will involve a comprehensive review of existing scholarly literature on cybersecurity in healthcare, machine learning applications in cybersecurity, and the specific threats targeting the healthcare sector. This will establish a strong theoretical foundation for the research and provide a detailed understanding of the current threat landscape, existing defense mechanisms, and the potential role of machine learning. Sources for this review will include academic databases (e.g., IEEE Xplore, ACM Digital Library, PubMed, Scopus), industry reports, and regulatory guidelines.
Phase 2: Identification and Evaluation of ML Techniques (Quantitative & Qualitative):
This phase will focus on identifying and evaluating machine learning algorithms and models that are most relevant to the identified cybersecurity threats in healthcare. This will involve:
- Quantitative Analysis: Experimenting with various supervised (e.g., classification algorithms like Support Vector Machines, Random Forests, Neural Networks) and unsupervised (e.g., anomaly detection algorithms like Isolation Forest, One-Class SVM, Clustering algorithms) machine learning models using publicly available or anonymized synthetic healthcare cybersecurity datasets (if access to real-world data is limited due to privacy concerns). The performance of these models will be evaluated based on metrics such as accuracy, precision, recall, F1-score, and detection rate.
- Qualitative Analysis: Conducting expert interviews with cybersecurity professionals, healthcare IT administrators, and data scientists with experience in both healthcare and machine learning. These interviews will aim to gather insights into the practical applicability, challenges, and perceived effectiveness of different ML techniques in real-world healthcare environments.
Phase 3: Case Studies and Implementation Challenges (Qualitative):
This phase will involve conducting case studies of healthcare organizations that have either successfully implemented or attempted to implement machine learning-based cybersecurity solutions. This qualitative approach will explore the organizational and technical considerations involved in the adoption process, including data integration, system compatibility, staff training, and addressing ethical and regulatory concerns (e.g., data privacy and bias in algorithms). Data will be collected through semi-structured interviews with relevant stakeholders and the analysis of organizational documents and reports (where accessible).
Phase 4: Development of a Conceptual Framework and Recommendations (Qualitative):
Drawing upon the findings from all preceding phases, this final phase will involve the development of a conceptual framework for the effective implementation of machine learning in healthcare cybersecurity. This framework will outline key considerations, best practices, and potential strategies for overcoming the identified challenges. The research will culminate in providing actionable recommendations for healthcare organizations seeking to enhance their cybersecurity posture through the strategic application of machine learning technologies.
Data Analysis:
Quantitative data from the machine learning model evaluations will be analyzed using statistical methods to compare the performance of different algorithms. Qualitative data from the literature review and interviews will be analyzed using thematic analysis to identify recurring patterns, key challenges, and expert opinions. The integration of both quantitative and qualitative findings will provide a richer and more nuanced understanding of the research problem.
Ethical Considerations:
Throughout the research process, strict adherence to ethical principles will be maintained. This includes ensuring the privacy and confidentiality of any data used (primarily relying on publicly available or anonymized synthetic data), obtaining informed consent from interview participants, and ensuring the responsible and unbiased application of machine learning techniques. The potential for bias in algorithms and the implications for data privacy in healthcare will be explicitly addressed in the analysis and recommendations.
This question has been answered.
Get AnswerOur Services
- Research Paper Writing
- Essay Writing
- Dissertation Writing
- Thesis Writing
Daily Statistics
- 134 New Projects
- 235 Projects in Progress
- 432 Inquiries
- 624 Repeat clients
Why Choose Us
- Money Return guarantee
- Guaranteed Privacy
- Written by Professionals
- Paper Written from Scratch
- Timely Deliveries
- Free Amendments